Yee Whye Teh
#6,974
Most Influential Person Now
Artificial intelligence researcher
Yee Whye Teh's AcademicInfluence.com Rankings
Yee Whye Tehcomputer-science Degrees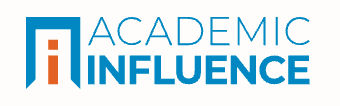
Computer Science
#383
World Rank
#398
Historical Rank
Machine Learning
#40
World Rank
#40
Historical Rank
Algorithms
#61
World Rank
#61
Historical Rank
Artificial Intelligence
#107
World Rank
#110
Historical Rank
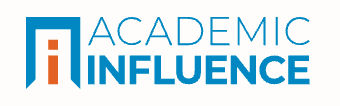
Download Badge
Computer Science
Yee Whye Teh's Degrees
- Bachelors Computer Science University of British Columbia
Similar Degrees You Can Earn
Why Is Yee Whye Teh Influential?
(Suggest an Edit or Addition)According to Wikipedia, Yee-Whye Teh is a professor of statistical machine learning in the Department of Statistics, University of Oxford. Prior to 2012 he was a reader at the Gatsby Charitable Foundation computational neuroscience unit at University College London. His work is primarily in machine learning, artificial intelligence, statistics and computer science.
Yee Whye Teh's Published Works
Number of citations in a given year to any of this author's works
Total number of citations to an author for the works they published in a given year. This highlights publication of the most important work(s) by the author
Published Works
- A Fast Learning Algorithm for Deep Belief Nets (2006) (14699)
- Hierarchical Dirichlet Processes (2006) (3732)
- Bayesian Learning via Stochastic Gradient Langevin Dynamics (2011) (2041)
- The Concrete Distribution: A Continuous Relaxation of Discrete Random Variables (2016) (1887)
- Set Transformer: A Framework for Attention-based Permutation-Invariant Neural Networks (2018) (644)
- Inferring the effectiveness of government interventions against COVID-19 (2020) (625)
- On Smoothing and Inference for Topic Models (2009) (605)
- A Hierarchical Bayesian Language Model Based On Pitman-Yor Processes (2006) (578)
- Progress & Compress: A scalable framework for continual learning (2018) (561)
- Do Deep Generative Models Know What They Don't Know? (2018) (551)
- A fast and simple algorithm for training neural probabilistic language models (2012) (549)
- Sharing Clusters among Related Groups: Hierarchical Dirichlet Processes (2004) (487)
- A Collapsed Variational Bayesian Inference Algorithm for Latent Dirichlet Allocation (2006) (486)
- Names and faces in the news (2004) (463)
- Distral: Robust multitask reinforcement learning (2017) (438)
- Conditional Neural Processes (2018) (419)
- Augmented Neural ODEs (2019) (390)
- Hierarchical Bayesian Nonparametric Models with Applications (2008) (313)
- Stochastic Gradient Riemannian Langevin Dynamics on the Probability Simplex (2013) (295)
- Dirichlet Process (2010) (293)
- Stick-breaking Construction for the Indian Buffet Process (2007) (287)
- Beam sampling for the infinite hidden Markov model (2008) (260)
- Semiparametric latent factor models (2005) (249)
- Attentive Neural Processes (2019) (245)
- Bayesian Nonparametrics: Hierarchical Bayesian nonparametric models with applications (2010) (231)
- Collapsed Variational Dirichlet Process Mixture Models (2007) (209)
- Consistency and Fluctuations For Stochastic Gradient Langevin Dynamics (2014) (208)
- Stacked Capsule Autoencoders (2019) (206)
- Sequential Attend, Infer, Repeat: Generative Modelling of Moving Objects (2018) (204)
- Automatic Alignment of Local Representations (2002) (198)
- Disentangling Disentanglement in Variational Autoencoders (2018) (189)
- Mondrian Forests: Efficient Online Random Forests (2014) (186)
- Energy-Based Models for Sparse Overcomplete Representations (2003) (185)
- Collapsed Variational Inference for HDP (2007) (181)
- Neural Processes (2018) (174)
- Bayesian Nonparametric Models (2010) (173)
- Filtering Variational Objectives (2017) (170)
- Continual Unsupervised Representation Learning (2019) (170)
- Tighter Variational Bounds are Not Necessarily Better (2018) (167)
- Rate-coded Restricted Boltzmann Machines for Face Recognition (2000) (165)
- Variational Inference for the Indian Buffet Process (2009) (154)
- A Bayesian Interpretation of Interpolated Kneser-Ney (2006) (152)
- The Mondrian Process (2008) (145)
- Unsupervised Discovery of Nonlinear Structure Using Contrastive Backpropagation (2006) (137)
- Bayesian Agglomerative Clustering with Coalescents (2007) (128)
- Belief Optimization for Binary Networks: A Stable Alternative to Loopy Belief Propagation (2001) (117)
- A stochastic memoizer for sequence data (2009) (117)
- Fast MCMC sampling for Markov jump processes and extensions (2012) (114)
- Continuous Hierarchical Representations with Poincaré Variational Auto-Encoders (2019) (113)
- MCMC for Normalized Random Measure Mixture Models (2013) (112)
- Indian Buffet Processes with Power-law Behavior (2009) (111)
- The Infinite Factorial Hidden Markov Model (2008) (110)
- Functional Regularisation for Continual Learning using Gaussian Processes (2019) (109)
- Probabilistic symmetry and invariant neural networks (2019) (105)
- Neural probabilistic motor primitives for humanoid control (2018) (102)
- AI for social good: unlocking the opportunity for positive impact (2020) (92)
- Exploration of the (Non-)Asymptotic Bias and Variance of Stochastic Gradient Langevin Dynamics (2016) (90)
- Meta reinforcement learning as task inference (2019) (90)
- Information asymmetry in KL-regularized RL (2019) (86)
- Approximate inference in Boltzmann machines (2003) (83)
- An Alternate Objective Function for Markovian Fields (2002) (82)
- Searching for objects driven by context (2012) (82)
- Detecting Out-of-Distribution Inputs to Deep Generative Models Using a Test for Typicality (2019) (79)
- Multiplicative Interactions and Where to Find Them (2020) (78)
- Task Agnostic Continual Learning via Meta Learning (2019) (77)
- DeepC: predicting 3D genome folding using megabase-scale transfer learning (2020) (75)
- Bayesian Rose Trees (2010) (75)
- Variational Bayesian Approach to Movie Rating Prediction (2007) (75)
- Variational Bayesian Optimal Experimental Design (2019) (75)
- Hybrid Models with Deep and Invertible Features (2019) (74)
- The sequence memoizer (2011) (72)
- The Unified Propagation and Scaling Algorithm (2001) (71)
- COIN: COmpression with Implicit Neural representations (2021) (71)
- An Analysis of Categorical Distributional Reinforcement Learning (2018) (71)
- Actor-Critic Reinforcement Learning with Energy-Based Policies (2012) (68)
- Making Latin Manuscripts Searchable using gHMMs (2004) (67)
- Improving Word Sense Disambiguation Using Topic Features (2007) (66)
- Distributed Bayesian Learning with Stochastic Natural Gradient Expectation Propagation and the Posterior Server (2015) (63)
- Gaussian Processes for Survival Analysis (2016) (62)
- Learning to Parse Images (1999) (61)
- Spatial Normalized Gamma Processes (2009) (60)
- NUS-ML:Improving Word Sense Disambiguation Using Topic Features (2007) (59)
- Bayesian Deep Ensembles via the Neural Tangent Kernel (2020) (59)
- Meta-learning of Sequential Strategies (2019) (59)
- Simple and Scalable Epistemic Uncertainty Estimation Using a Single Deep Deterministic Neural Network (2020) (58)
- LieTransformer: Equivariant self-attention for Lie Groups (2020) (57)
- Generative Models as Distributions of Functions (2021) (56)
- The effectiveness and perceived burden of nonpharmaceutical interventions against COVID-19 transmission: a modelling study with 41 countries (2020) (56)
- Bayesian nonparametric crowdsourcing (2014) (56)
- Distributed Bayesian Posterior Sampling via Moment Sharing (2014) (56)
- Mix&Match - Agent Curricula for Reinforcement Learning (2018) (55)
- A Statistical Approach to Assessing Neural Network Robustness (2018) (54)
- Bayesian nonparametric Plackett-Luce models for the analysis of preferences for college degree programmes (2012) (52)
- Poisson Random Fields for Dynamic Feature Models (2016) (51)
- Structured Region Graphs: Morphing EP into GBP (2005) (50)
- Mondrian Forests for Large-Scale Regression when Uncertainty Matters (2015) (49)
- Hamiltonian Descent Methods (2018) (47)
- Fast MCMC sampling for Markov jump processes and continuous time Bayesian networks (2011) (47)
- The effectiveness of eight nonpharmaceutical interventions against COVID-19 in 41 countries (2020) (47)
- A Hierarchical Nonparametric Bayesian Approach to Statistical Language Model Domain Adaptation (2009) (46)
- Bayesian multi-population haplotype inference via a hierarchical dirichlet process mixture (2006) (46)
- Dependent Dirichlet Process Spike Sorting (2008) (46)
- Causal Inference via Kernel Deviance Measures (2018) (46)
- Asynchronous Anytime Sequential Monte Carlo (2014) (45)
- MetaFun: Meta-Learning with Iterative Functional Updates (2019) (45)
- Scaling up the Automatic Statistician: Scalable Structure Discovery using Gaussian Processes (2017) (43)
- Gaussian process modulated renewal processes (2011) (43)
- Linear Response Algorithms for Approximate Inference in Graphical Models (2004) (43)
- Riemannian Score-Based Generative Modeling (2022) (43)
- Relativistic Monte Carlo (2016) (40)
- Neural Ensemble Search for Uncertainty Estimation and Dataset Shift (2020) (40)
- Concave-Convex Adaptive Rejection Sampling (2011) (39)
- Modelling Genetic Variations using Fragmentation-Coagulation Processes (2011) (39)
- Exploiting Hierarchy for Learning and Transfer in KL-regularized RL (2019) (38)
- Infinite Hierarchical Hidden Markov Models (2009) (38)
- Dependent Normalized Random Measures (2013) (38)
- Lossless Compression Based on the Sequence Memoizer (2010) (37)
- Poisson intensity estimation with reproducing kernels (2016) (37)
- On Improving the Efficiency of the Iterative Proportional Fitting Procedure (2003) (36)
- Fractional Underdamped Langevin Dynamics: Retargeting SGD with Momentum under Heavy-Tailed Gradient Noise (2020) (36)
- Stochastic Expectation Maximization with Variance Reduction (2018) (35)
- Revisiting Reweighted Wake-Sleep for Models with Stochastic Control Flow (2018) (35)
- Neural Ensemble Search for Performant and Calibrated Predictions (2020) (35)
- Inferring ground truth from multi-annotator ordinal data: a probabilistic approach (2013) (34)
- An Efficient Sequential Monte Carlo Algorithm for Coalescent Clustering (2008) (33)
- MCMC for continuous-time discrete-state systems (2012) (33)
- Bayesian nonparametric models for ranked data (2012) (33)
- Discovering Multiple Constraints that are Frequently Approximately Satisfied (2001) (31)
- A Unified Stochastic Gradient Approach to Designing Bayesian-Optimal Experiments (2019) (30)
- Faithful Inversion of Generative Models for Effective Amortized Inference (2017) (29)
- DR-ABC: Approximate Bayesian Computation with Kernel-Based Distribution Regression (2016) (28)
- Top-down particle filtering for Bayesian decision trees (2013) (28)
- Dirichlet Processes: Tutorial and Practical Course (2007) (27)
- Hybrid Variational/Gibbs Collapsed Inference in Topic Models (2008) (26)
- Improvements to the Sequence Memoizer (2010) (25)
- Set Transformer (2018) (25)
- Behavior Priors for Efficient Reinforcement Learning (2020) (24)
- The Mondrian Kernel (2016) (23)
- Powerpropagation: A sparsity inducing weight reparameterisation (2021) (23)
- Robust Pruning at Initialization (2020) (23)
- A New View of ICA (2001) (22)
- Faces and names in the news (2004) (22)
- Revisiting Reweighted Wake-Sleep (2018) (22)
- Bayesian Hierarchical Community Discovery (2013) (21)
- Particle Gibbs for Bayesian Additive Regression Trees (2015) (20)
- Expectation Particle Belief Propagation (2015) (20)
- Hierarchical Representations with Poincaré Variational Auto-Encoders (2019) (19)
- Understanding the 2016 US Presidential Election using ecological inference and distribution regression with census microdata (2016) (19)
- Making Forward Chaining Relevant (1998) (19)
- Lottery Tickets in Linear Models: An Analysis of Iterative Magnitude Pruning (2020) (18)
- Rediscovery of Good–Turing estimators via Bayesian nonparametrics (2014) (18)
- COIN++: Data Agnostic Neural Compression (2022) (18)
- Pre-training via Denoising for Molecular Property Prediction (2022) (18)
- On the Stick-Breaking Representation for Homogeneous NRMIs (2016) (17)
- Bayesian Nonparametrics for Sparse Dynamic Networks (2016) (17)
- How Robust are the Estimated Effects of Nonpharmaceutical Interventions against COVID-19? (2020) (17)
- Names and faces. (1985) (17)
- Learning Label Trees for Probabilistic Modelling of Implicit Feedback (2011) (17)
- Detecting Out-of-Distribution Inputs to Deep Generative Models Using Typicality (2019) (17)
- Modelling sparsity, heterogeneity, reciprocity and community structure in temporal interaction data (2018) (16)
- Bootstrapping Neural Processes (2020) (16)
- A Marginal Sampler for σ-Stable Poisson–Kingman Mixture Models (2014) (15)
- Particle Value Functions (2017) (15)
- Deep Amortized Clustering (2019) (14)
- Scalable imputation of genetic data with a discrete fragmentation-coagulation process (2012) (14)
- Pruning untrained neural networks: Principles and Analysis (2020) (13)
- Scaled subordinators and generalizations of the Indian buffet process (2015) (13)
- DeepC: Predicting chromatin interactions using megabase scaled deep neural networks and transfer learning (2019) (13)
- Mixed Cumulative Distribution Networks (2010) (13)
- Meta-Learning surrogate models for sequential decision making (2019) (13)
- Challenges and Opportunities in Offline Reinforcement Learning from Visual Observations (2022) (13)
- Random Tessellation Forests (2019) (13)
- Equivariant Learning of Stochastic Fields: Gaussian Processes and Steerable Conditional Neural Processes (2020) (13)
- Bayesian nonparametric inference for discovery probabilities: credible intervals and large sample asymptotics (2015) (13)
- Hierarchical Dirichlet Trees for Information Retrieval (2009) (12)
- Discovering Nonbinary Hierarchical Structures with Bayesian Rose Trees (2011) (12)
- names and faces. (1982) (12)
- Noise Contrastive Meta-Learning for Conditional Density Estimation using Kernel Mean Embeddings (2019) (12)
- Divide, Conquer, and Combine: a New Inference Strategy for Probabilistic Programs with Stochastic Support (2019) (12)
- Inference Trees: Adaptive Inference with Exploration (2018) (11)
- Bethe free energy and contrastive divergence approximations for undirected graphical models (2003) (11)
- Nonexchangeable random partition models for microclustering (2017) (11)
- Multi-Armed Bandit for Species Discovery: A Bayesian Nonparametric Approach (2018) (10)
- Dual Control (2010) (10)
- Dual Space Preconditioning for Gradient Descent (2019) (9)
- Random variate generation for Laguerre-type exponentially tilted $\alpha$-stable distributions (2015) (9)
- Stick-breaking construction for the Indian buffet (2007) (9)
- Interoperability of statistical models in pandemic preparedness: principles and reality. (2021) (9)
- Group Equivariant Subsampling (2021) (9)
- Bayesian nonparametric Plackett-Luce models for the analysis of clustered ranked data (2012) (9)
- Cooled and Relaxed Survey Propagation for MRFs (2007) (8)
- Efficient Bayesian inference of instantaneous reproduction numbers at fine spatial scales, with an application to mapping and nowcasting the Covid‐19 epidemic in British local authorities (2022) (8)
- Vector-valued Gaussian Processes on Riemannian Manifolds via Gauge Independent Projected Kernels (2021) (8)
- Meta-Learning Sparse Compression Networks (2022) (7)
- BayesIMP: Uncertainty Quantification for Causal Data Fusion (2021) (7)
- The Mondrian Process for Machine Learning (2015) (7)
- Modeling Population Structure Under Hierarchical Dirichlet Processes (2015) (7)
- Controllable Semantic Image Inpainting (2018) (7)
- Linear Response for Approximate Inference (2003) (7)
- A mixture model for the evolution of gene expression in non-homogeneous datasets (2008) (6)
- Statistical Verification of Neural Networks (2018) (6)
- Conformal Off-Policy Prediction in Contextual Bandits (2022) (6)
- A hybrid sampler for Poisson-Kingman mixture models (2015) (6)
- A characterization of product-form exchangeable feature probability functions (2016) (6)
- On Incorporating Inductive Biases into VAEs (2021) (6)
- COIN++: Neural Compression Across Modalities (2022) (6)
- Collaborative Filtering with Side Information: a Gaussian Process Perspective (2016) (5)
- names and faces. (1982) (5)
- Transferring Task Goals via Hierarchical Reinforcement Learning (2018) (5)
- Continual Learning via Sequential Function-Space Variational Inference (2022) (5)
- Image Retrieval with a Bayesian Model of Relevance Feedback (2016) (5)
- Scalable Structure Discovery in Regression using Gaussian Processes (2016) (5)
- On Exploration, Exploitation and Learning in Adaptive Importance Sampling (2018) (5)
- Functional Regularisation for Continual Learning (2019) (5)
- Variational Optimal Experiment Design : Efficient Automation of Adaptive Experiments (2018) (5)
- Learning with Invariance via Linear Functionals on Reproducing Kernel Hilbert Space (2013) (5)
- On Contrastive Representations of Stochastic Processes (2021) (5)
- Sampling and Inference for Beta Neutral-to-the-Left Models of Sparse Networks (2018) (5)
- Bayesian Inference with Big Data : A Snapshot from a Workshop (2014) (5)
- Deep Kernel Machines via the Kernel Reparametrization Trick (2017) (4)
- UncertaINR: Uncertainty Quantification of End-to-End Implicit Neural Representations for Computed Tomography (2022) (4)
- Amortized Rejection Sampling in Universal Probabilistic Programming (2019) (4)
- Variational Estimators for Bayesian Optimal Experimental Design (2019) (4)
- Technical Document 3: E↵ectiveness and Resource Requirements of Test, Trace and Isolate Strategies (2020) (4)
- Decision Stump (2010) (4)
- Neural network models of exchangeable sequences (2018) (3)
- On a class of $$\sigma $$σ-stable Poisson–Kingman models and an effective marginalized sampler (2015) (3)
- Importance Weighted Policy Learning and Adaption (2020) (3)
- Hijacking Malaria Simulators with Probabilistic Programming (2019) (3)
- Adaptive Reconfiguration Moves for Dirichlet Mixtures (2014) (3)
- Tractable Function-Space Variational Inference in Bayesian Neural Networks (2022) (3)
- Attentive Clustering Processes (2020) (3)
- Semi-supervised Learning in Reproducing Kernel Hilbert Spaces Using Local Invariances (2006) (3)
- Posterior Consistency for a Non-parametric Survival Model under a Gaussian Process Prior (2016) (3)
- Bayesian Inference on Population Structure: From Parametric to Nonparametric Modeling (2015) (2)
- Abstract B2-59: PhyloSpan: Using multi-mutation reads to resolve subclonal architectures from heterogeneous tumor samples (2015) (2)
- Equivariant Conditional Neural Processes (2020) (2)
- On the stick-breaking representation of $\sigma$-stable Poisson-Kingman models (2014) (2)
- GCNU TR 2001 – 001 Passing And Bouncing Messages For Generalized Inference (2001) (2)
- Tucker Gaussian Process for Regression and Collaborative Filtering (2016) (2)
- A nonparametric HMM for genetic imputation and coalescent inference (2016) (2)
- Bias Variance Decomposition (2010) (2)
- An Exact Auxiliary Variable Gibbs Sampler for a Class of Diffusions (2019) (2)
- NEVIS'22: A Stream of 100 Tasks Sampled from 30 Years of Computer Vision Research (2022) (2)
- When Does Re-initialization Work? (2022) (2)
- On Big Data Learning for Small Data Problems (2018) (2)
- Sampling and inference for discrete random probability measures in probabilistic programs (2017) (2)
- Non-exchangeable feature allocation models with sublinear growth of the feature sizes (2020) (2)
- Bellman Equation (2010) (1)
- Instance-Specific Augmentation: Capturing Local Invariances (2022) (1)
- InteL-VAEs: Adding Inductive Biases to Variational Auto-Encoders via Intermediary Latents (2021) (1)
- Amortized Probabilistic Detection of Communities in Graphs (2020) (1)
- Infinite Hidden Markov Models via the Hierarchical Dirichlet Process (2005) (1)
- Guest Editors' Introduction to the Special Issue on Bayesian Nonparametrics (2015) (1)
- Appendices for Tighter Variational Bounds are Not Necessarily Better (2018) (1)
- Incorporating Unlabelled Data into Bayesian Neural Networks (2023) (1)
- Bayesian Nonparametrics Rough Notes (2012) (1)
- A Tractable Class of σ-stable Poisson-Kingman Processes and an Effective Marginalized Sampler (2014) (1)
- Unsupervised Object Discovery via Capsule Decoders (2019) (1)
- On Statistical Thinking in Deep Learning (2019) (1)
- On the robustness of effectiveness estimation of nonpharmaceutical interventions against COVID-19 transmission (2020) (1)
- Bayesian Nonparametric Modeling and the Ubiquitous Ewens Sampling Formula (2016) (1)
- Bayesian tools for natural language learning (2011) (1)
- An Introduction to Bayesian Nonparametric Modelling (2009) (1)
- epidemics/COVIDNPIs: Inferring the effectiveness of government interventions against COVID-19 (2020) (1)
- Dec . 7 , 2018 Critiquing and Correcting Trends in Machine Learning (0)
- Learning Instance-Specific Data Augmentations (2022) (0)
- Inference in Boltzmann Machines, Mean Field, TAP and Bethe Approximations (2007) (0)
- Minimally Faithful Inversion of Graphical Models (2017) (0)
- Deep Transformers without Shortcuts: Modifying Self-attention for Faithful Signal Propagation (2023) (0)
- Uncertainty Quantification in End-to-End Implicit Neural Representations for Medical Imaging (2021) (0)
- Stick-breaking representations of 1/2-stable Poisson-Kingman models (2013) (0)
- On Bayesian nonparametric inference for discovery probabilites (2016) (0)
- Danish Sound Technology Network Intelligent Sound Center for Computational Cognitive Modeling (2009) (0)
- Supplementary Material for Set Transformer (2019) (0)
- Abstract 4865: PhyloSpan: using multi-mutation reads to resolve subclonal architectures from heterogeneous tumor samples (2015) (0)
- Faithful Model Inversion Substantially Improves Auto-encoding Variational Inference (2017) (0)
- neural compression workshop COIN : CO MPRESSION WITH I MPLICIT N EURAL REPRESENTATIONS (2021) (0)
- Vector-valued Gaussian Processes on Riemannian Manifolds via Gauge Equivariant Projected Kernels (2021) (0)
- Distribution-Free Learning (2010) (0)
- (Invited talk) Bayesian Tools for Natural Language Learning (2011) (0)
- Hierarchical Dririchlet tree for information retrieval (2009) (0)
- Supplementary Material to "Cooled and Relaxed Survey Propagation for MRFs" (2007) (0)
- A TTENTIVE N EURAL P ROCESSES (2019) (0)
- Approximate inference by Markov chains on union spaces (2004) (0)
- Riemannian Diffusion Schr\"odinger Bridge (2022) (0)
- Mix & Match – Agent Curricula for Reinforcement Learning [ Appendix ] (2018) (0)
- TR A 2 / 0 6 A Bayesian Interpretation of Interpolated Kneser (2004) (0)
- names and faces. (1982) (0)
- Infinitely Deep Infinite-Width Networks (2018) (0)
- Hierarchical Dirichlet Processes Author ( s ) : (2011) (0)
- Supplementary Material for Bootstrapping Neural Processes (2020) (0)
- NUS-ML:Improving Word Sense Disambiguation Using Topic Features (2007) (0)
- Constructing dependent random probability measures from completely random measures (2013) (0)
- Ecient Laplace kernel approximation with a Mondrian process (2009) (0)
- Spatial Variations in COVID-19 Transmission Rates in UK (2020) (0)
- for Normalized Random Measure Mixture Models (2013) (0)
- Convex Algorithms in Approximate Inference (2009) (0)
- Daisee: Adaptive Importance Sampling by Balancing Exploration and Exploitation (2023) (0)
- On a class of σ -stable Poisson–Kingman models and an effective marginalized sampler (2015) (0)
- Latent Variable Models for Time Series (2010) (0)
- Thompson sampling for species discovery (2016) (0)
- Proceedings of the Workshop on Prior Knowledge for Text and Language Processing (2008) (0)
- Modality-Agnostic Variational Compression of Implicit Neural Representations (2023) (0)
- Adaptive Reconfiguration Moves for MCMC inference in Dirichlet Process Mixtures (2014) (0)
This paper list is powered by the following services:
Other Resources About Yee Whye Teh
What Schools Are Affiliated With Yee Whye Teh?
Yee Whye Teh is affiliated with the following schools: