Cynthia Rudin
American computer scientist and statistician
Cynthia Rudin's AcademicInfluence.com Rankings
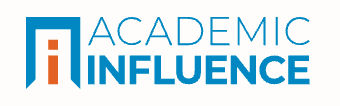
Download Badge
Computer Science Mathematics
Cynthia Rudin's Degrees
- PhD Statistics Princeton University
- Masters Statistics Princeton University
- Bachelors Mathematics University of Chicago
Similar Degrees You Can Earn
Why Is Cynthia Rudin Influential?
(Suggest an Edit or Addition)According to Wikipedia, Cynthia Diane Rudin is an American computer scientist and statistician specializing in machine learning and known for her work in interpretable machine learning. She is the director of the Interpretable Machine Learning Lab at Duke University, where she is a professor of computer science, electrical and computer engineering, statistical science, and biostatistics and bioinformatics. In 2022, she won the Squirrel AI Award for Artificial Intelligence for the Benefit of Humanity from the Association for the Advancement of Artificial Intelligence for her work on the importance of transparency for AI systems in high-risk domains.
Cynthia Rudin's Published Works
Published Works
- Stop explaining black box machine learning models for high stakes decisions and use interpretable models instead (2018) (3026)
- Interpretable classifiers using rules and Bayesian analysis: Building a better stroke prediction model (2015) (621)
- This looks like that: deep learning for interpretable image recognition (2018) (605)
- All Models are Wrong, but Many are Useful: Learning a Variable's Importance by Studying an Entire Class of Prediction Models Simultaneously (2018) (544)
- Deep Learning for Case-based Reasoning through Prototypes: A Neural Network that Explains its Predictions (2017) (357)
- PULSE: Self-Supervised Photo Upsampling via Latent Space Exploration of Generative Models (2020) (310)
- Supersparse linear integer models for optimized medical scoring systems (2015) (264)
- The Bayesian Case Model: A Generative Approach for Case-Based Reasoning and Prototype Classification (2014) (259)
- The Big Data Newsvendor: Practical Insights from Machine Learning (2019) (246)
- Machine Learning for the New York City Power Grid (2012) (228)
- Falling Rule Lists (2014) (225)
- Interpretable Machine Learning: Fundamental Principles and 10 Grand Challenges (2021) (205)
- Interpretable classification models for recidivism prediction (2015) (200)
- Learning Certifiably Optimal Rule Lists for Categorical Data (2017) (184)
- The World Health Organization Adult Attention-Deficit/Hyperactivity Disorder Self-Report Screening Scale for DSM-5 (2017) (181)
- Please Stop Explaining Black Box Models for High Stakes Decisions (2018) (181)
- Arabic Morphological Tagging, Diacritization, and Lemmatization Using Lexeme Models and Feature Ranking (2008) (176)
- Why Are We Using Black Box Models in AI When We Don’t Need To? A Lesson From An Explainable AI Competition (2019) (166)
- Scalable Bayesian Rule Lists (2016) (165)
- Model Class Reliance: Variable Importance Measures for any Machine Learning Model Class, from the "Rashomon" Perspective (2018) (162)
- A Bayesian Framework for Learning Rule Sets for Interpretable Classification (2017) (160)
- The P-Norm Push: A Simple Convex Ranking Algorithm that Concentrates at the Top of the List (2009) (160)
- Learning to Detect Patterns of Crime (2013) (153)
- Concept whitening for interpretable image recognition (2020) (153)
- All Models are Wrong but many are Useful: Variable Importance for Black-Box, Proprietary, or Misspecified Prediction Models, using Model Class Reliance (2018) (120)
- The Dynamics of AdaBoost: Cyclic Behavior and Convergence of Margins (2004) (117)
- The age of secrecy and unfairness in recidivism prediction (2018) (116)
- Optimal Sparse Decision Trees (2019) (115)
- Learning classification models of cognitive conditions from subtle behaviors in the digital Clock Drawing Test (2015) (110)
- A Shared Vision for Machine Learning in Neuroscience (2018) (109)
- Margin-based Ranking and an Equivalence between AdaBoost and RankBoost (2009) (109)
- Learning Certifiably Optimal Rule Lists (2017) (104)
- Machine learning for science and society (2013) (92)
- Association of an Electroencephalography-Based Risk Score With Seizure Probability in Hospitalized Patients (2017) (89)
- Optimized Scoring Systems: Toward Trust in Machine Learning for Healthcare and Criminal Justice (2018) (87)
- Margin-Based Ranking Meets Boosting in the Middle (2005) (83)
- Understanding How Dimension Reduction Tools Work: An Empirical Approach to Deciphering t-SNE, UMAP, TriMAP, and PaCMAP for Data Visualization (2020) (83)
- An Interpretable Model with Globally Consistent Explanations for Credit Risk (2018) (77)
- Clinical Prediction Models for Sleep Apnea: The Importance of Medical History over Symptoms. (2016) (75)
- Learning Cost-Effective and Interpretable Treatment Regimes (2017) (73)
- Ranking with a P-Norm Push (2006) (72)
- A study in Rashomon curves and volumes: A new perspective on generalization and model simplicity in machine learning (2019) (71)
- Interpretable Image Recognition with Hierarchical Prototypes (2019) (65)
- Generalized and Scalable Optimal Sparse Decision Trees (2020) (61)
- A process for predicting manhole events in Manhattan (2010) (61)
- Online coordinate boosting (2008) (60)
- The Rate of Convergence of Adaboost (2011) (60)
- An Interpretable Stroke Prediction Model using Rules and Bayesian Analysis (2013) (56)
- Algorithms for interpretable machine learning (2014) (55)
- Bayesian Rule Sets for Interpretable Classification (2016) (54)
- Sequential Event Prediction with Association Rules (2011) (54)
- Reactive point processes: A new approach to predicting power failures in underground electrical systems (2015) (52)
- A Hierarchical Model for Association Rule Mining of Sequential Events: An Approach to Automated Medical Symptom Prediction (2011) (52)
- Optimized Risk Scores (2017) (51)
- A case-based interpretable deep learning model for classification of mass lesions in digital mammography (2021) (50)
- Machine learning with operational costs (2011) (50)
- Bayesian Hierarchical Rule Modeling for Predicting Medical Conditions (2012) (46)
- Sequential event prediction (2013) (46)
- Learning customized and optimized lists of rules with mathematical programming (2018) (44)
- Learning theory analysis for association rules and sequential event prediction (2013) (43)
- Methods and Models for Interpretable Linear Classification (2014) (43)
- Discovery with Data: Leveraging Statistics with Computer Science to Transform Science and Society (2014) (42)
- Box drawings for learning with imbalanced data (2014) (42)
- Learning Optimized Risk Scores (2016) (41)
- Supersparse Linear Integer Models for Interpretable Classification (2013) (40)
- Or's of And's for Interpretable Classification, with Application to Context-Aware Recommender Systems (2015) (39)
- Analysis of boosting algorithms using the smooth margin function (2007) (37)
- The Big Data Newsvendor: Practical Insights from Machine Learning Analysis (2013) (34)
- Building Interpretable Classifiers with Rules using Bayesian Analysis (2012) (34)
- FLAME: A Fast Large-scale Almost Matching Exactly Approach to Causal Inference (2017) (33)
- Robust Optimization using Machine Learning for Uncertainty Sets (2014) (33)
- An optimization approach to learning falling rule lists (2017) (32)
- On Equivalence Relationships Between Classification and Ranking Algorithms (2011) (30)
- Learning to Predict the Wisdom of Crowds (2012) (28)
- Detecting Patterns of Crime with Series Finder (2013) (28)
- Finding Patterns with a Rotten Core: Data Mining for Crime Series with Cores (2015) (27)
- Re-Ranking Algorithms for Name Tagging (2006) (26)
- Boosting Based on a Smooth Margin (2004) (26)
- Analytics for Power Grid Distribution Reliability in New York City (2014) (25)
- On combining machine learning with decision making (2011) (25)
- The Secrets of Machine Learning: Ten Things You Wish You Had Known Earlier to be More Effective at Data Analysis (2019) (23)
- Machine Learning Algorithms for Classification (2007) (23)
- In Pursuit of Interpretable, Fair and Accurate Machine Learning for Criminal Recidivism Prediction (2020) (21)
- Globally-Consistent Rule-Based Summary-Explanations for Machine Learning Models: Application to Credit-Risk Evaluation (2019) (21)
- Exploring the cloud of variable importance for the set of all good models (2020) (20)
- An Integer Optimization Approach to Associative Classification (2012) (20)
- Supersparse Linear Integer Models for Predictive Scoring Systems (2013) (20)
- MALTS: Matching After Learning to Stretch (2018) (20)
- Approximating the crowd (2014) (19)
- Growing a list (2013) (18)
- Causal Falling Rule Lists (2015) (18)
- Prediction uncertainty and optimal experimental design for learning dynamical systems. (2015) (18)
- ORC: Ordered Rules for ClassificationA Discrete Optimization Approach to Associative Classification (2012) (18)
- Variable Importance Clouds: A Way to Explore Variable Importance for the Set of Good Models (2019) (17)
- Data quality assurance and performance measurement of data mining for preventive maintenance of power grid (2011) (17)
- Learning Optimized Or's of And's (2015) (17)
- A Theory of Statistical Inference for Ensuring the Robustness of Scientific Results (2018) (17)
- Evaluating Machine Learning for Improving Power Grid Reliability (2011) (16)
- On the Dynamics of Boosting (2003) (16)
- Metaphor Detection Using Contextual Word Embeddings From Transformers (2020) (16)
- How to reverse-engineer quality rankings (2012) (16)
- Learning about meetings (2013) (15)
- Interpretable Almost-Exact Matching for Causal Inference (2019) (15)
- New Techniques for Preserving Global Structure and Denoising with Low Information Loss in Single-Image Super-Resolution (2018) (15)
- Causal Rule Sets for Identifying Subgroups with Enhanced Treatment Effect (2017) (15)
- Approximating the Wisdom of the Crowd (2011) (14)
- Towards Practical Lipschitz Bandits (2019) (13)
- Reducing Noise in Labels and Features for a Real World Dataset: Application of NLP Corpus Annotation Methods (2009) (13)
- Bayesian Inference of Arrival Rate and Substitution Behavior from Sales Transaction Data with Stockouts (2015) (13)
- Tire Changes, Fresh Air, and Yellow Flags: Challenges in Predictive Analytics for Professional Racing (2014) (13)
- Interpretable, not black-box, artificial intelligence should be used for embryo selection (2021) (13)
- A Bayesian Approach to Learning Scoring Systems (2015) (12)
- The Machine Learning and Traveling Repairman Problem (2011) (12)
- On the Existence of Simpler Machine Learning Models (2019) (12)
- 21st-Century Data Miners Meet 19th-Century Electrical Cables (2011) (12)
- Precise Statements of Convergence for AdaBoost and arc-gv (2007) (11)
- Interpretable Machine Learning Models for the Digital Clock Drawing Test (2016) (11)
- Adaptive Hyper-box Matching for Interpretable Individualized Treatment Effect Estimation (2020) (11)
- A Transformer Approach to Contextual Sarcasm Detection in Twitter (2020) (11)
- Learning Optimized Risk Scores on Large-Scale Datasets (2016) (11)
- Estimation of System Reliability Using a (2011) (10)
- A Holistic Approach to Interpretability in Financial Lending: Models, Visualizations, and Summary-Explanations (2021) (10)
- Towards a comprehensive evaluation of dimension reduction methods for transcriptomic data visualization (2022) (10)
- The latent state hazard model, with application to wind turbine reliability (2015) (10)
- Almost-Matching-Exactly for Treatment Effect Estimation under Network Interference (2020) (9)
- Estimation of system reliability using a semiparametric model (2011) (9)
- Machine Learning and the Traveling Repairman (2011) (9)
- Integer Optimization Methods for Supervised Ranking (2011) (8)
- Ethical Implementation of Artificial Intelligence to Select Embryos in In Vitro Fertilization (2021) (8)
- The Factorized Self-Controlled Case Series Method: An Approach for Estimating the Effects of Many Drugs on Many Outcomes (2016) (8)
- Interpretable Mammographic Image Classification using Cased-Based Reasoning and Deep Learning (2021) (8)
- Direct Learning to Rank and Rerank (2018) (8)
- A Computational Model of Inhibition of HIV-1 by Interferon-Alpha (2015) (8)
- Hypothesis Tests That Are Robust to Choice of Matching Method (2018) (8)
- A supervised machine learning semantic segmentation approach for detecting artifacts in plethysmography signals from wearables (2021) (7)
- Modeling recovery curves with application to prostatectomy. (2015) (6)
- Predicting Power Failures with Reactive Point Processes (2013) (6)
- Preference Learning (Dagstuhl Seminar 14101) (2014) (6)
- Learning Cost-Effective Treatment Regimes using Markov Decision Processes (2016) (6)
- A Learning Theory Framework for Association Rules and Sequential Events (2011) (5)
- Stability Analysis for Regularized Least Squares Regression (2005) (5)
- Why black box machine learning should be avoided for high-stakes decisions, in brief (2022) (5)
- Interpretable Almost Matching Exactly With Instrumental Variables (2019) (5)
- SegDiscover: Visual Concept Discovery via Unsupervised Semantic Segmentation (2022) (5)
- Learning Cost-Effective and Interpretable Regimes for Treatment Recommendation (2016) (5)
- Do Simpler Models Exist and How Can We Find Them? (2019) (5)
- Failure Analysis of the New York City Power Grid (2014) (5)
- Fast Sparse Decision Tree Optimization via Reference Ensembles (2021) (5)
- A Minimax Surrogate Loss Approach to Conditional Difference Estimation (2018) (5)
- Towards Practical Lipschitz Stochastic Bandits. (2019) (5)
- A Different Type of Convergence for Statistical Learning Algorithms (2003) (5)
- Report Cards for Manholes: Eliciting Expert Feedback for a Learning Task (2009) (5)
- Visualization of Manhole and Precursor-Type Events for the Manhattan Electrical Distribution System (2008) (5)
- Regulating Greed Over Time (2015) (4)
- AI reflections in 2019 (2020) (4)
- Reducing Exploration of Dying Arms in Mortal Bandits (2019) (4)
- Wisely Using a Budget for Crowdsourcing (2012) (4)
- Treatment Effect of Repairs to an Electrical Grid Leveraging a Machine Learned Model of Structure Vulnerability (2011) (4)
- Bandits for BMO Functions (2020) (4)
- Regulating Greed Over Time in Multi-Armed Bandits (2021) (4)
- Multitask Learning for Citation Purpose Classification (2021) (4)
- Effects of Epileptiform Activity on Discharge Outcome in Critically Ill Patients: A Retrospective Cross-Sectional Study (2022) (4)
- A Practical Risk Score for EEG Seizures in Hospitalized Patients (S11.002) (2018) (3)
- The Importance of Being Ernest, Ekundayo, or Eswari: An Interpretable Machine Learning Approach to Name-Based Ethnicity Classification (2022) (3)
- Progressive Clustering with Learned Seeds: An Event Categorization System for Power Grid (2012) (3)
- CRAFT: ClusteR-specific Assorted Feature selecTion (2015) (3)
- A Learning Theory Framework for Sequential Event Prediction and Association Rules (2012) (3)
- Generalized Optimal Sparse Decision Trees (2020) (3)
- Fast Sparse Classification for Generalized Linear and Additive Models (2022) (3)
- Boosting, margins, and dynamics (2004) (3)
- Bayesian Patchworks: An Approach to Case-Based Reasoning (2018) (3)
- Extreme Dimension Reduction for Handling Covariate Shift (2017) (3)
- Interpretable Almost Matching Exactly for Causal Inference (2018) (3)
- Simple Rules for Predicting Congestion Risk in Queueing Systems: Application to ICUs (2019) (3)
- Playing Codenames with Language Graphs and Word Embeddings (2021) (3)
- How Smart Guessing Strategies Can Yield Massive Scalability Improvements for Sparse Decision Tree Optimization (2021) (3)
- Generalization bounds for learning with linear, polygonal, quadratic and conic side knowledge (2014) (3)
- Exploring the Whole Rashomon Set of Sparse Decision Trees (2022) (3)
- BacHMMachine: An Interpretable and Scalable Model for Algorithmic Harmonization for Four-part Baroque Chorales (2021) (3)
- Predicting Manhole Events in Manhattan A Case Study in Extended Knowledge Discovery (2009) (3)
- There Once Was a Really Bad Poet, It Was Automated but You Didn’t Know It (2021) (2)
- Selective Sampling of Labelers for Approximating the Crowd (2012) (2)
- Learning classification models of cognitive conditions from subtle behaviors in the digital Clock Drawing Test (2015) (2)
- Open Problem: Does AdaBoost Always Cycle? (2012) (2)
- dame-flame: A Python Library Providing Fast Interpretable Matching for Causal Inference (2021) (2)
- Shall I Compare Thee to a Machine-Written Sonnet? An Approach to Algorithmic Sonnet Generation (2018) (2)
- Latent Case Model: A Generative Approach for Case-Based Reasoning and Prototype Classification (2014) (2)
- How to See Hidden Patterns in Metamaterials with Interpretable Machine Learning (2021) (2)
- Report Cards for Manholes (2009) (2)
- FasterRisk: Fast and Accurate Interpretable Risk Scores (2022) (2)
- Rethinking Nonlinear Instrumental Variable Models through Prediction Validity (2022) (2)
- Modeling Weather Impact on a Secondary Electrical Grid (2014) (2)
- Learning Cost-Effective and Interpretable Treatment Regimes for Judicial Bail Decisions (2016) (2)
- Prediction, Machine Learning, and Individual Lives: an Interview with Matthew Salganik (2020) (2)
- TimberTrek: Exploring and Curating Sparse Decision Trees with Interactive Visualization (2022) (2)
- Cascaded High Dimensional Histograms: A Generative Approach to Density Estimation (2015) (2)
- Almost-Exact Matching with Replacement for Causal Inference (2018) (2)
- Matching Bounds: How Choice of Matching AlgorithmImpacts Treatment Effects Estimates and What to Do about It (2020) (1)
- Machine Learning for Meeting Analysis (2013) (1)
- Statistical Thinking and Data Analysis (PDF) (2011) (1)
- Predicting Vulnerability to Serious Manhole Events In Manhattan : A Preliminary Machine Learning Approach (2008) (1)
- A Robust Approach to Quantifying Uncertainty in Matching Problems of Causal Inference (2018) (1)
- Dimension Reduction for Robust Covariate Shift Correction (2017) (1)
- On combining machine learning with decision making (2014) (1)
- Broader Issues Surrounding Model Transparency in Criminal Justice Risk Scoring (2020) (1)
- Erratum: "Prediction uncertainty and optimal experimental design for learning dynamical systems" [Chaos 26, 063110 (2016)]. (2017) (1)
- A Practical Bandit Method with Advantages in Neural Network Tuning (2019) (1)
- A phase I study of dose-dense “alternating doublets” chemotherapy with filgrastim (rhG-CSF) support in advanced non-small cell lung cancer (NSCLC) (2000) (1)
- Cryo-ZSSR: multiple-image super-resolution based on deep internal learning (2020) (1)
- Interpretable Almost-Exact Matching for Causal Inference Supplementary Material (2019) (1)
- Robust Testing for Causal Inference in Observational Studies (2015) (1)
- Fast optimization of weighted sparse decision trees for use in optimal treatment regimes and optimal policy design (2022) (1)
- Cascaded High Dimensional Histograms: An Approach to Interpretable Density Estimation for Categorical Data (2015) (0)
- Generalization Bounds for Learning with Linear and Quadratic Side Knowledge (2014) (0)
- USING THE DIGITAL CLOCK DRAWING TEST AND MACHINE LEARNING TO IMPROVE ACCURACY OF COGNITIVE SCREENING (2016) (0)
- Foundations of Sequential Learning (2018) (0)
- A Statistical Learning Theory Framework for Supervised Pattern Discovery (2013) (0)
- J un 2 01 4 Box Drawings for Learning with Imbalanced Data (2014) (0)
- There is no Accuracy-Interpretability Tradeoff in Reinforcement Learning for Mazes (2022) (0)
- Learning about meetings (2014) (0)
- Machine learning for science and society (2013) (0)
- ‘ ‘ A KEY DIFFERENCE BETWEEN PREDICTIVE MODELING FOR PROFESSIONAL RACING COMPARED TO THAT IN BASKETBALL ( AND BASEBALL ) IS THE NATURE OF THE EVOLUTION OF THE GAME (2014) (0)
- Learning customized and optimized lists of rules with mathematical programming (2018) (0)
- Matched Machine Learning: A Generalized Framework for Treatment Effect Inference With Learned Metrics (2023) (0)
- Growing a list (2013) (0)
- Sequential Event Prediction with Association Rules Citation (2011) (0)
- Glass Box Artificial Intelligence in Criminal Justice (2022) (0)
- Approximating the Crowd SUPPLEMENTARY MATERIAL (2014) (0)
- Introduction to Stochastic Multi-Armed Bandits (2020) (0)
- Direct Learning to Rank and Rerank 1 (2018) (0)
- Pre-trial Risk Analysis (2018) (0)
- OKRidge: Scalable Optimal k-Sparse Ridge Regression for Learning Dynamical Systems (2023) (0)
- OPERATIONS RESEARCH CENTER Working Paper MASSACHUSETTS INSTITUTE OF TECHNOLOGY by Bayesian Hierarchical Rule Modeling for Predicting Medical Conditions OR 385-11 (2011) (0)
- USING THE DIGITAL CLOCK DRAWING TEST AND MACHINE LEARNING TO IMPROVE ACCURACY OF COGNITIVE SCREENING (2016) (0)
- Teaching \Prediction: Machine Learning and Statistics" (2012) (0)
- Causal Inference with High-Dimensional Controls and Parameters of Interest (2017) (0)
- Interpretable deep learning models for better clinician-AI communication in clinical mammography (2022) (0)
- How to reverse-engineer quality rankings (2012) (0)
- Generalization bounds for learning with linear, polygonal, quadratic and conic side knowledge (2014) (0)
- Sequential event prediction (2013) (0)
- Collapsing-Fast-Large-Almost-Matching-Exactly: A Matching Method for Causal Inference (2018) (0)
- Supersparse linear integer models for optimized medical scoring systems (2015) (0)
- Approximating the crowd (2014) (0)
- PULSE: Supplementary Material (2020) (0)
- Submitted to the Annals of Statistics ANALYSIS OF BOOSTING ALGORITHMS USING THE SMOOTH MARGIN FUNCTION ∗ By (2007) (0)
- Interpretable Matching for Causal Inference [R package FLAME version 2.0.0] (2020) (0)
- Supplement to Growing a List (2013) (0)
- Direct Learning to Rank and Rerank : Supplementary File AISTATS 2018 (2018) (0)
- Toward a Theory of Pattern Discovery (2014) (0)
- From Feature Importance to Distance Metric: An Almost Exact Matching Approach for Causal Inference (2023) (0)
- Why Interpretable Causal Inference is Important for High-Stakes Decision Making for Critically Ill Patients and How To Do It (2022) (0)
- The Influence of Operational Cost on Estimation (2012) (0)
- Why Interpretable Causal Inference is Important for High-Stakes Medical Decision Making in Neurology and How to Do it (2022) (0)
- Optimal Sparse Regression Trees (2022) (0)
- Missing Values and Imputation in Healthcare Data: Can Interpretable Machine Learning Help? (2023) (0)
- Data solidarity for machine learning for embryo selection: a call for the creation of an open access repository of embryo data. (2022) (0)
- Understanding and Exploring the Whole Set of Good Sparse Generalized Additive Models (2023) (0)
- Data poisoning attacks on off-policy policy evaluation methods (2022) (0)
- Stop explaining black box machine learning models for high stakes decisions and use interpretable models instead (2019) (0)
- Embryo Selection by “Black-Box” Artificial Intelligence: The Ethical and Epistemic Considerations (2022) (0)
- O-098 Embryo selection using Artificial Intelligence (AI): Epistemic and ethical considerations (2021) (0)
This paper list is powered by the following services:
Other Resources About Cynthia Rudin
What Schools Are Affiliated With Cynthia Rudin?
Cynthia Rudin is affiliated with the following schools: